Monetizing AI: Business Models That Work (And Those That Don’t)
✍️ Opinions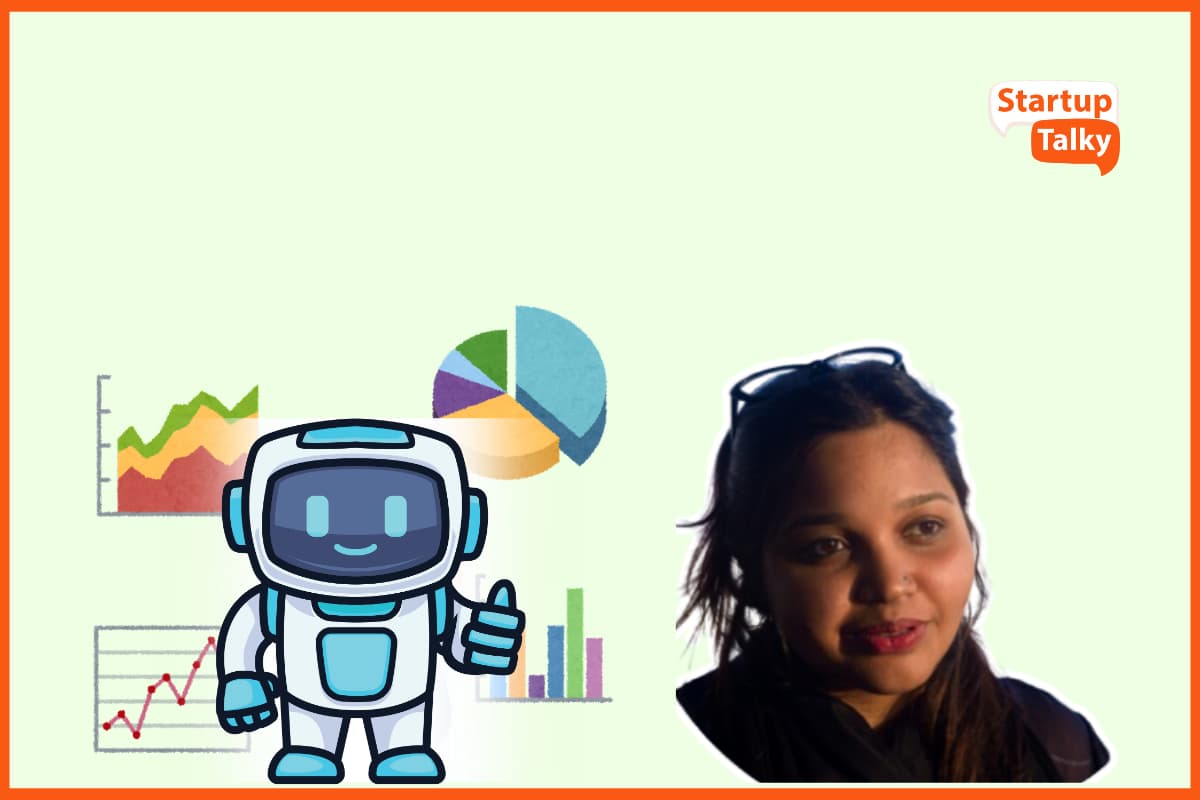
This article has been contributed by Nida Sahar, CEO, Nife.io.
In 2017, a small AI startup had what seemed like an unbeatable product: a powerful deep-learning model that could generate human-like text. Investors were excited, the demo was impressive, and the hype was real. But there was a major challenge—they had no clear monetization strategy.
After months of experimentation, they launched a subscription product, but user churn was high. They then attempted to license their model to enterprises, but the sales cycle was too slow. Finally, they pivoted to an API model, which allowed them to scale revenue quickly.
That startup was OpenAI.
Their journey reflects a challenge every AI company faces: Having powerful technology is not enough—without the right business model, even the most advanced AI products will fail.
Three Proven AI Monetization Strategies
AI startups typically choose among three primary monetization strategies, each with advantages and challenges.
1. Subscription-Based AI: The SaaS Model
A common approach to AI applications is the subscription-based SaaS model. Whether it’s an AI-powered tool for marketing, automation, or data analysis, many companies opt to charge users a monthly or annual subscription.
When Subscription Works Best
- The product provides continuous value (e.g., Grammarly enhances writing daily).
- A freemium-to-premium conversion strategy is effective (Jasper AI monetized free users by offering premium marketing features).
- The target audience consists of individuals or small businesses that are unlikely to commit to enterprise contracts.
Challenges of Subscription AI
- High churn rates: If users do not perceive ongoing value, retention becomes difficult.
- Customer acquisition costs: Scaling an AI SaaS product requires significant investment in marketing and customer education.
- Compute costs: AI-driven SaaS products often have higher infrastructure costs than traditional SaaS, which can eat into margins.
Case Study: Grammarly & Jasper AI
Grammarly successfully leveraged the freemium model, allowing users to test the product before upgrading to paid plans. Jasper AI found a profitable niche in marketing, charging users based on AI-generated content.
Both succeeded because they solved a specific, recurring pain point rather than simply offering an interesting AI feature.
2. API-Based AI: The Developer-Focused Approach
Instead of building an end-user application, some AI startups monetize by offering API access to their models, allowing developers and businesses to integrate AI into their own products.
When APIs Work Best
- Developers need off-the-shelf AI without the cost of in-house development. ● The AI model is expensive to build but relatively cheap to scale (Deepgram optimized infrastructure to make speech recognition cost-effective).
- Enterprises prefer usage-based pricing over fixed subscriptions.
Challenges of API Monetization
- Cloud costs can escalate if you don't carefully structure pricing; usage growth can lead to unsustainable infrastructure costs.
- Commoditization risk: Open-source alternatives and competitors can drive prices down, leading to a race to the bottom.
Case Study: OpenAI & Deepgram
OpenAI successfully implemented pay-per-use pricing, which allowed it to generate significant revenue while maintaining accessibility. Deepgram positioned itself as a cost-effective alternative to major cloud providers by optimizing infrastructure and pricing aggressively.
APIs are scalable, but success depends on controlling costs and maintaining differentiation in a competitive market.
3. Enterprise AI: Selling to Large Organizations
Enterprise AI focuses on selling AI solutions directly to businesses, often through customized deployments or large-scale integrations. This model is common in industries like finance, healthcare, cybersecurity, and government contracting.
When Enterprise AI Works Best
- The AI product solves a critical business problem that organizations cannot solve internally (e.g., Palantir provides intelligence solutions to government agencies).
- Customers require custom AI models that are not easily replaced by off-the-shelf solutions.
- The company has the resources to withstand long sales cycles (often 12+ months).
Challenges of Enterprise AI
- Sales cycles are long: Closing enterprise deals can take a year or more, creating cash flow challenges for early-stage startups.
- Customer acquisition requires direct sales efforts, which can be expensive and complex.
- Procurement processes are slow and bureaucratic, making it difficult to scale quickly. Case Study: Palantir & C3.ai
Palantir built a successful AI business by securing large government contracts before expanding into the private sector. C3.ai focused on industry-specific AI applications, such as energy and supply chain optimization, allowing them to differentiate from general-purpose AI platforms.
Enterprise AI can be highly profitable, but it requires significant capital, patience, and a strong sales team.
What Doesn’t Work?
While some AI business models have proven successful, others have consistently failed.
1. “Cool Tech Without a Business Model”
Many AI startups focus too much on research and product development without a clear go-to-market strategy. Having a high-performing model is not enough; it needs to be packaged, priced, and distributed effectively.
2. “Subscription Models with High Churn”
If users do not see continuous value from an AI product, they will cancel their subscriptions quickly. AI tools that are used sporadically or fail to integrate into users’ workflows often struggle to retain customers.
3. “APIs Without Pricing Control”
APIs can be profitable, but only if usage-based pricing accounts for infrastructure costs. If an API model scales usage without sufficient margins, the company can end up losing money as it grows.
4. “Enterprise AI Without Sufficient Funding”
Many startups attempt to sell AI to enterprises without realizing how long and expensive the process is. Without strong financial backing, these companies often run out of capital before closing enough deals to sustain operations.
Pricing AI Products: Key Strategies
Selecting the right pricing model is critical for AI monetization. The most successful companies use one or a combination of the following approaches:
1. Usage-Based Pricing (Best for APIs & Enterprise AI)
- Charges customers per API call, token processed, or data analyzed.
- Example: OpenAI’s pricing scales based on usage.
2. Tiered Subscription Pricing (Best for SaaS AI)
- Offers multiple pricing plans based on feature access or limits.
- Example: Jasper AI charges higher fees for businesses, generating more AI content.
3. Freemium-to-Paid Conversion (Best for Consumer AI)
- Provides free access to basic features, with paid upgrades for advanced functionality.
- Example: Grammarly’s free version drives user adoption before upselling premium features.
4. Enterprise Licensing (Best for B2B AI)
- Companies sell AI solutions as a one-time license or an annual contract.
- Example: Palantir’s multi-million-dollar government contracts.
Key Takeaways for AI Founders and Investors
- Subscription AI can work, but retention is critical.
- API-first models scale quickly, but pricing and cloud costs must be managed carefully.
- Enterprise AI is lucrative but requires capital, patience, and strong sales execution.
- A hybrid approach often provides the most stability and scalability. What’s Your Strategy?
The AI market is exploding, but monetization remains a major challenge. The most successful AI companies are not necessarily those with the best technology, but those that have a well-defined business model and execution plan.
For AI founders, the key question is not just, “What can we build?” but “How will we sell it?”.
Must have tools for startups - Recommended by StartupTalky
- Convert Visitors into Leads- SeizeLead
- Website Builder SquareSpace
- Manage your business Smoothly Google Business Suite