5 Financial Analysis Terms Every Startup Data Professional Needs to Know
impact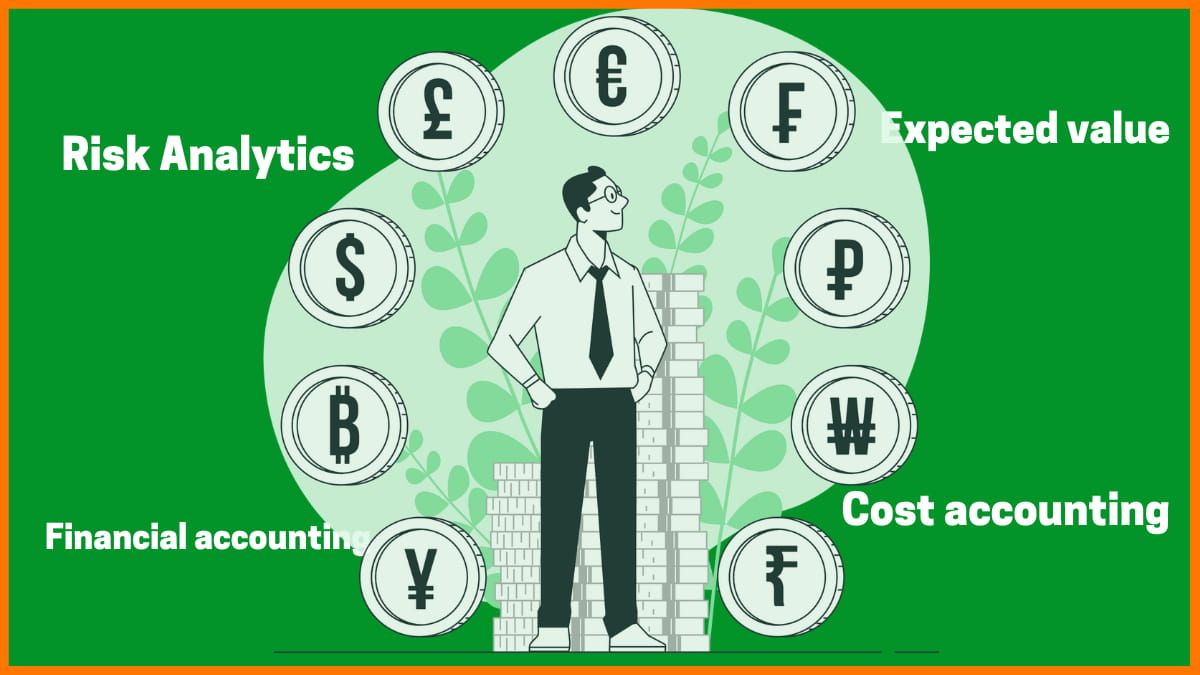
When it comes to in-house talent at startups, the best data scientists have one important factor that sets them apart: they are interested in and knowledgeable about the overall business picture of the company where they work.
It doesn’t matter if you’re operating in the mobile app sector, running a DTC ecommerce brand, or any other business vertical – you can be as skilled as you like at building and deploying algorithms, but unless you understand how they apply to your company’s bottom line, you won’t get far.
Not only that, but as big data becomes a tool in every part of startup operations, many data scientists may find their talents and interests begin aligning with a realm of niche expertise such as security, customer service, sales, or marketing. One of the most exciting new areas for data scientists is in the financial sector, as financial analysts are beginning to see the rewards of incorporating typical data science skills like artificial intelligence into their jobs and day-to-day tasks.
Even if you’re not working in the finance sector, financial data is an increasingly important part of the picture, and financial tasks become part of the job. This is the case for any industry you’re in – the FP&A team is likely to inevitably need help with data reporting analysis.
No matter where your career path will take you, data scientists should at least be able to understand these five basic financial analysis terms to continue at the top of their game and keep themselves open to opportunities in their own field or in a new one.
1. Risk analytics
Risk management is an important part of any team at a growing startup. Typically, it falls to the C-suite. But as companies grow more adept at collecting and sorting through data, risk analytics has gained prominence. Previously, leaders would use their intuition to guide their ventures. Today, there has to be hard data behind their decisions.
The purpose of risk analytics is to “measure, quantify, and even predict risk,” according to Deloitte. It requires data scientists to build models that will monitor, assess, and report risk to the relevant parties.
It’s not just a finance department responsibility, either – risk assessment has to create a unified view of all sorts of risks the company faces, and how to approach them from a data-backed perspective.
2. Expected value
In statistics, expected value has a pretty well-defined meaning: it’s simply the anticipated average value for an investment, calculated by multiplying each of the possible outcomes by the likelihood that each outcome will occur. Then, these values are all summed up.
In the finance world, these values allow investors to choose the scenario that is most likely to give them the most desired outcome. In the data science world, the expected value is one of the underpinning concepts of probability theory and machine learning. When an executive is wondering whether she should take that big risk or not, a data scientist will likely be called in to discuss what the long-term outcome is most likely to be.
Many data scientists will know the mathematical definition and significance, but it pays to be aware of the relevance of the term in a business sense, too.
3. Cost accounting
Cost accounting is the process by which a company will identify how it is spending money, how much money it’s earning, and where money is being lost.
An example of this is cost volume profit analysis, where FP&A leaders will determine how many sales it would take to break even with the cost of doing business. Simply put, it’s a way to hypothetically balance the books.
Data scientists are being called in to help with this process, since it involves a holistic look at the business’s expenses and potential earnings across multiple departments. To create a realistic cost accounting model, data scientists need a good grasp of their employer’s business model.
4. Financial accounting
Financial accounting is retrospective – it’s an analysis of transactions that have occurred over a past period of time. Despite the similarity of the name, financial accounting is subtly different from cost accounting.
Cost accounting is geared towards internal audiences. However, financial accounting is outward-facing. It’s designed to help shareholders, lenders, and regulators get a better view of the business. The statements presented in financial accounting show revenues, expenses, assets, liabilities, and equity.
Accounting is by its very nature an analytical skill. As data science languages and techniques have evolved, it’s become more efficient to use data science skills to create reports and plans of financial accounting.
5. Credit allocation
Credit allocation is one of the secretive forces behind economic growth. It’s defined by Mathur and Marcelin as “a process of how a bank divides its financial resources and other sources of credit to different processes, borrowers and projects.” Basically, it’s how sources of credit decide how much financial credit to give people.
This goes hand in hand with the risk analytics discussed earlier. Data scientists frequently are in the position of building models that will help decide how much credit to allocate, like credit scores. Data scientists are needed to help companies optimize profit while minimizing risk to themselves.
Credit allocation is an enormous topic, especially if you’re unfamiliar. To learn more about it, I recommend starting with an actual case study. This paper from 2017 examines the relationship between credit allocation, risk management, and loan portfolio performance of microfinance institutions. It’s a fascinating read, and data scientists can follow along with the methodology to understand how their own skills can be useful.
These financial terms are just stepping stones
This is a short article, and each of these terms has had multiple books written on the subject. Think of this as more of an introductory guide than a definitive source of knowledge.
Whether you plan to move into the finance sector or not, data scientists need to have a thorough knowledge of financial terms and concepts to stay competitive in the workforce. These five are not comprehensive, but they’re a good place to start.
Must have tools for startups - Recommended by StartupTalky
- Convert Visitors into Leads- SeizeLead
- Manage your business smoothly- Google Workspace
- International Money transfer- XE Money Transfer